The Rise of Agentic AI and its RPA Roots
In the ever-evolving landscape of technology, the concept of agentic AI has emerged as a buzzword, capturing the attention of both tech giants and startups alike. With the shift away from smaller language models, the focus has turned toward implementing AI agents within various workflows. However, as NVIDIA CEO Jensen Huang suggested, the future of IT departments might resemble more of an HR role for these AI agents, a notion echoed by Microsoft’s Satya Nadella, who envisioned a swarm of AI agents in the workforce.
RPA’s Unfulfilled Promise
The comparison to Robotic Process Automation (RPA) is an intriguing one. RPA was predicted to automate mundane tasks, allowing teams to focus on more complex projects. Yet, this promise remained largely unfulfilled. Now, industry experts draw parallels between the current hype around AI agents and the RPA phase, raising questions about their true capabilities and potential.
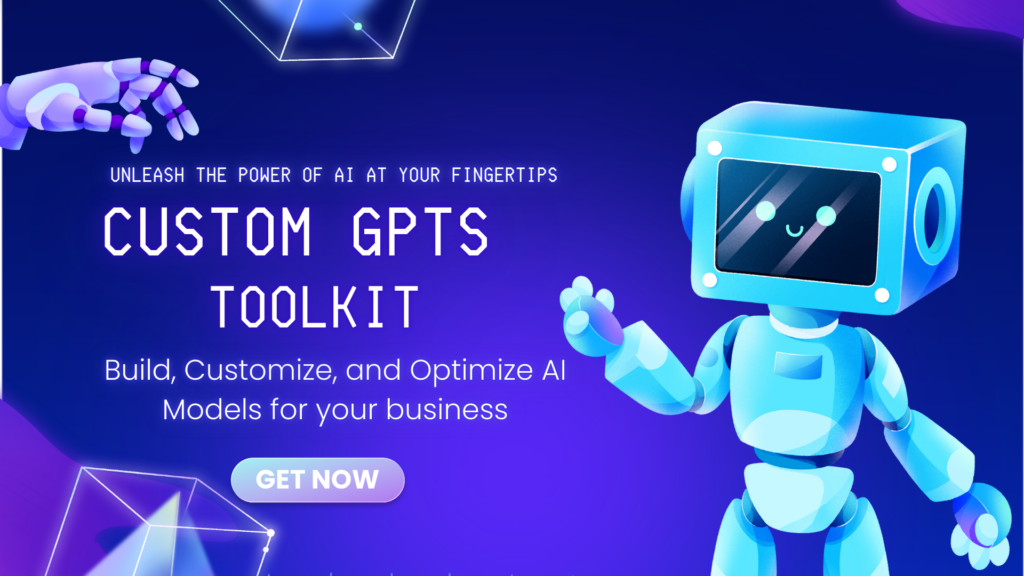
What is RPA?
Robotic Process Automation (RPA) is a technology that uses software robots or “bots” to automate repetitive, rule-based tasks typically performed by humans. These tasks often involve interacting with digital systems and applications, mimicking human actions like clicking, typing, and navigating interfaces.
Key Features of RPA:
- Automation of Routine Tasks: RPA handles repetitive processes such as data entry, form filling, and report generation.
- Non-Invasive Integration: RPA tools work on top of existing systems without requiring significant changes to the underlying software.
- Scalability: Bots can be scaled up or down depending on the workload.
- Rule-Based Execution: RPA follows predefined workflows and business rules, making it suitable for structured tasks.
Benefits of RPA:
- Increased Efficiency: Bots work faster and more accurately than humans, leading to productivity gains.
- Cost Savings: Automating routine tasks reduces the need for human labor, saving operational costs.
- Error Reduction: Bots eliminate the risk of human errors in tasks like data processing.
- Improved Compliance: RPA ensures consistent adherence to rules and regulations.
Common Applications:
- Data Migration and Entry: Moving data between systems.
- Invoice Processing: Automating accounts payable workflows.
- Customer Support: Resolving simple customer queries using bots.
- Report Generation: Compiling and formatting data into reports.
Limitations:
- Rigid Processes: RPA struggles with unstructured data or scenarios that require judgment.
- Maintenance Overhead: Changes in underlying applications may require bots to be updated.
- No Learning Capability: Traditional RPA lacks adaptability without AI or machine learning enhancements.
With advancements in AI and machine learning, many RPA tools are evolving to incorporate cognitive capabilities, transforming into more sophisticated solutions like Agentic AI, which adds reasoning and adaptability to automation.
Agentic Loops and their Implications
Nikhil Malhotra, Chief Innovation Officer at Tech Mahindra, highlights that while many startups will talk about agentic AI, the underlying technology might just be an enhanced version of RPA. The key difference, he suggests, lies in the agentic loops these startups are beginning to consider. For instance, Anthropic’s Claude 3.5 Sonnet, with its ability to interact with software programs and navigate websites, showcases an agentic approach that blurs the lines between RPA and AI agents.
The LLM Factor
The integration of Large Language Models (LLMs) is a game-changer. It transforms the traditional RPA framework into something more sophisticated and potentially revolutionary. As Malhotra puts it, “the good thing” about this evolution is that it encourages startups to think beyond the limitations of RPA, exploring the possibilities of agentic loops.
A Market Shift?
The predicted shift from the $250 billion SaaS market to a $300 billion AI agents market is a bold claim. Companies adopting AI agents in their workflows might revolutionize the way we work, but the price difference and the unconvincing nature of this transition raise valid concerns. Moreover, the entry of established RPA companies into the AI agent race further blurs the lines between the two technologies.
RPA’s Evolution or a New Era?
Param Kahlon, EVP and GM of Automation and Integration at Salesforce emphasizes that autonomous agents do not signal the end of RPA technology. Instead, he views RPA agents as tools designed for specific, repetitive tasks, while autonomous agents process information more like humans, adapting to changing conditions and making informed decisions. This shift, according to Ramprakash Ramamoorthy, Director of AI Research at ManageEngine and Zoho, opens a new era of self-directed operations, enabling faster scaling and better responses to evolving business needs.
The Transformative Power of Agentic AI
Agentic AI is more than just RPA with LLMs; it represents a transformative evolution. While traditional RPA executes predefined tasks, agentic AI learns, reasons, and adapts in real time, adding a layer of cognitive flexibility to process automation. Shailesh, the tech YouTuber, describes the majority of agentic applications as essentially workflow automation with minimal human interaction, cautioning against the overhyped notion of autonomy.
Beyond RPA: The Power of Decision Trees
Anil Kumar, CTO at Exotel, draws a compelling analogy, stating that calling agentic AI “RPA with LLMs” is as unconvincing as saying C++ classes are just C structures with methods. He explains that while RPA deals with structured data, agentic AI aims to interpret decision trees, a more complex and nuanced approach. Taking the example of loan negotiations, Kumar highlights how agentic AI navigates the intricacies of human conversation, carrying context, previous learnings, and contractual constraints to make informed decisions.
The Evolution of AI Integration in RPA
Between 2018 and 2023, the integration of AI into RPA solutions has steadily enhanced its functionality. However, it was the emergence of agentic AI in 2024 that marked a true breakthrough. Andreessen Horowitz’s thesis predicts that AI will automate operations, potentially rendering traditional RPA obsolete.
The Tactical vs. Goal-Based Approach
Deepak Dastrala, CTO and Partner at IntellectAI emphasizes the tactical nature of RPA, which focuses on automating repetitive, rule-based tasks. In contrast, AI agents take a more goal-based approach, acting as digital twins of humans, powered by LLMs, and equipped with memory and real-time learning capabilities. This shift, according to Dastrala, is why RPA’s relevance has faded, while AI agents are poised to revolutionize our work in ways no other automation technology has achieved.
The Future of Agentic AI
Arnav Bathla, CEO of Layerup, predicts the end of the era of cargo cult programming, suggesting that in ten years, RPA and agent studios will be relics of the past. Instead, he envisions a future where specialized agents, uniquely designed for specific industries, solve problems end-to-end.
Conclusion: RPA 2.0 or a New Paradigm?
Agentic AI can be viewed as an enhanced version of RPA, or RPA 2.0, with the addition of LLMs. However, the rebranding of advanced RPA as agentic AI is often a marketing strategy to capitalize on AI’s hype. The fundamental objective of automating repetitive tasks for efficiency remains unchanged. Yet, the fate of agentic AI might depend on its ability to evolve and address its limitations, ensuring it doesn’t meet the same fate as RPA.
Download n8n Template of Automated Kids Story (Free download)
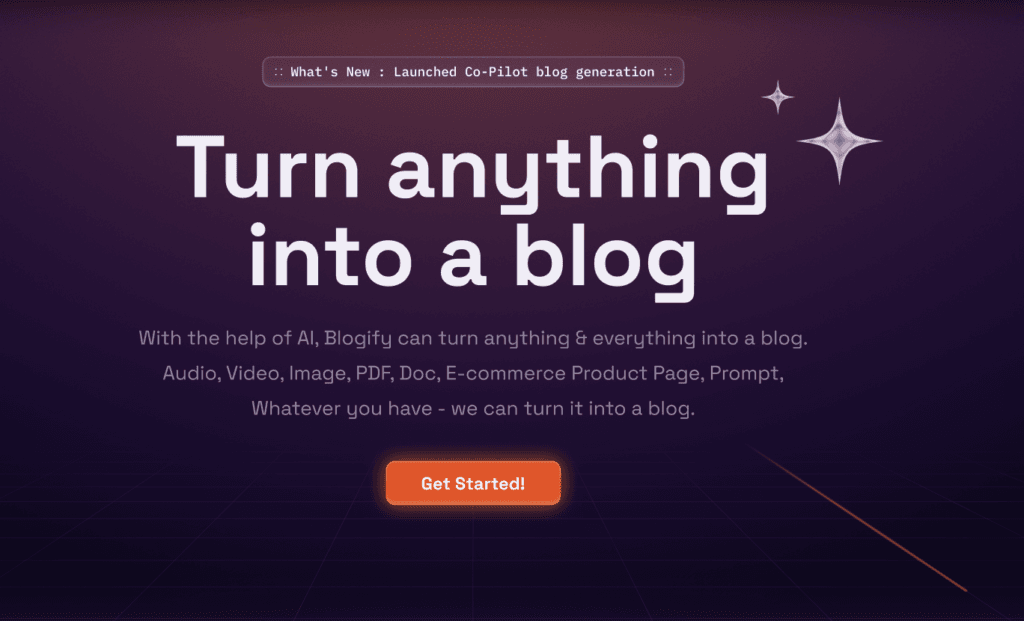